In today’s data-driven world, gathering and analyzing news trends is crucial for businesses to stay ahead of the competition. This blog will guide you through creating data gathering pipelines to collect news trends from various sources, storing the data in a data warehouse, and integrating this process with a large language model (LLM) using Python and langchain.
Step 1: Set Up Your Environment
Before we start, ensure you have Python installed on your machine. We will also need several Python libraries, including requests
, pandas
, sqlalchemy
, and langchain
. You can install these using pip:
pip install requests pandas sqlalchemy langchain
Step 2: Gathering News Data
We will use the requests
library to gather news data from various sources. For this example, we’ll gather data from a hypothetical news API.
Create a Python Script to Collect News Data
Create a new Python file named news_data_gathering.py
and add the following code:
import requests
import pandas as pd
from datetime import datetime
def fetch_news(api_url, api_key):
response = requests.get(api_url, headers={'Authorization': f'Bearer {api_key}'})
if response.status_code == 200:
return response.json()
else:
print(f"Failed to fetch news: {response.status_code}")
return []
def parse_news_data(news_json):
articles = news_json.get('articles', [])
news_data = []
for article in articles:
news_data.append({
'title': article.get('title', ''),
'description': article.get('description', ''),
'url': article.get('url', ''),
'published_at': article.get('publishedAt', ''),
'source': article.get('source', {}).get('name', '')
})
return pd.DataFrame(news_data)
def main():
api_url = "https://api.example.com/news"
api_key = "your_api_key"
news_json = fetch_news(api_url, api_key)
news_df = parse_news_data(news_json)
news_df.to_csv(f"news_data_{datetime.now().strftime('%Y%m%d')}.csv", index=False)
if __name__ == "__main__":
main()
This script fetches news data from a specified API, parses the JSON response, and saves it as a CSV file.
Step 3: Storing Data in a Data Warehouse
Next, we will store the collected data in a data warehouse using sqlalchemy
.
Create a Python Script to Store Data in a Data Warehouse
Create a new Python file named store_news_data.py
and add the following code:
import pandas as pd
from sqlalchemy import create_engine
def load_news_data(csv_file):
return pd.read_csv(csv_file)
def store_in_data_warehouse(df, db_url):
engine = create_engine(db_url)
df.to_sql('news_trends', con=engine, if_exists='append', index=False)
def main():
csv_file = "news_data_20240101.csv" # Replace with your CSV file name
db_url = "postgresql://username:password@localhost:5432/mydatabase"
news_df = load_news_data(csv_file)
store_in_data_warehouse(news_df, db_url)
if __name__ == "__main__":
main()
This script reads the CSV file generated in the previous step and stores the data in a PostgreSQL data warehouse.
Step 4: Integrating with langchain and LLM
To integrate this process with a large language model using langchain, we will create a script that combines the data gathering and storage processes and interacts with the LLM.
Create a Python Script to Integrate with LLM
Create a new Python file named integrate_with_llm.py
and add the following code:
from langchain import LangChain
import news_data_gathering
import store_news_data
def main():
# Step 1: Gather news data
news_data_gathering.main()
# Step 2: Store data in the data warehouse
store_news_data.main()
# Step 3: Integrate with LLM
llm = LangChain(api_key="your_langchain_api_key")
query = "Analyze the latest business news trends."
# Assuming the LLM can access the data warehouse directly
result = llm.query_database(query)
print("LLM Analysis Result:", result)
if __name__ == "__main__":
main()
This script automates the data gathering and storage processes and queries the LLM for analysis. Ensure to replace placeholders like your_langchain_api_key
with your actual API keys and credentials.
Conclusion
In this blog, we’ve walked through setting up data gathering pipelines to collect news trends, storing the data in a data warehouse, and integrating the process with a large language model using Python and langchain. This setup provides a robust framework for continuously collecting, storing, and analyzing news trends, enabling businesses to make data-driven decisions.
Feel free to customize the scripts and integrate additional data sources as needed. If you want to build a pipeline similar to this, get in touch with DataStunt. We specialize in creating data pipelines, data warehousing solutions, and integrating advanced analytics with machine learning models to help you get the most out of your data.
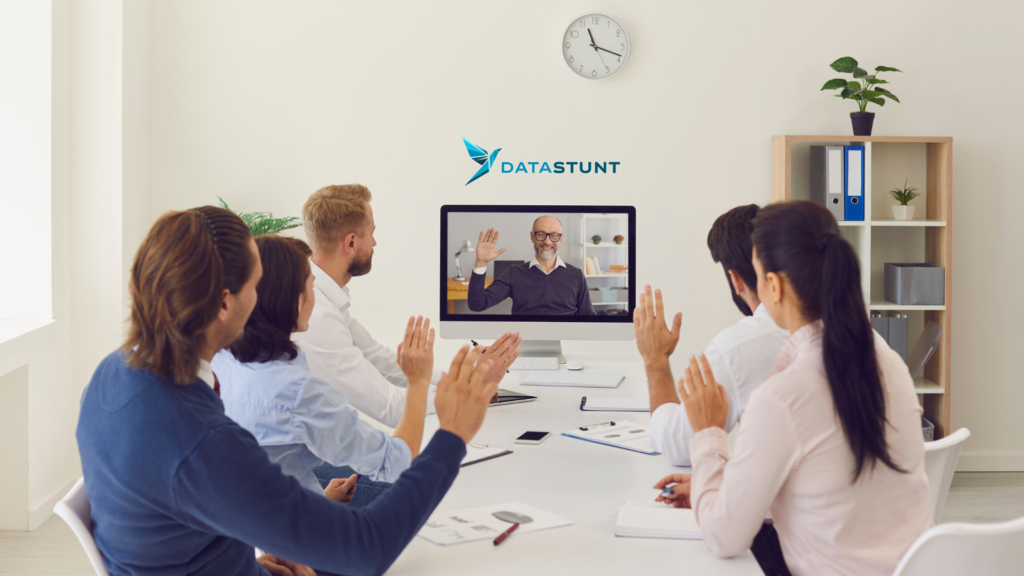
Happy coding!
Thanks forr sharing such a pleasant thought, piecce off writing is fastidious,
thats why i have read it entirely https://Evolution.ORG.Ua/